What is Quantitative Trading?
Want to know about quantitative trading? Let’s know everything about what is quantitive, and how it works. Let’s get a detailed and its various types on besuremoney.
What exactly is Quantitative Trading?
Quantitative Trading uses quantitative analysis. It relies on math to navigate the stock market. Quantitative trading is also known as quant trading. This discipline uses statistics and probability. It has changed how traders navigate markets. These funds are non-traditional and considered passive.
It relies on statistical calculations and numerical decomposition to identify trading opportunities. Price and volume are the two most common data types in math models. They are key for quantitative analysis.
Financial institutions and hedge funds often use volume trading. The trades are usually large. It may include buying and selling hundreds of thousands of shares and other securities.
Why quantitative trading has gained popularity in financial markets?
It relies on data and statistics. Statistical analysts use it to make investment decisions. Algorithms identify market patterns and predict trends. They let quantitative marketers seize opportunities that traditional marketers may miss.
What are the basic concepts of quantitative trading?
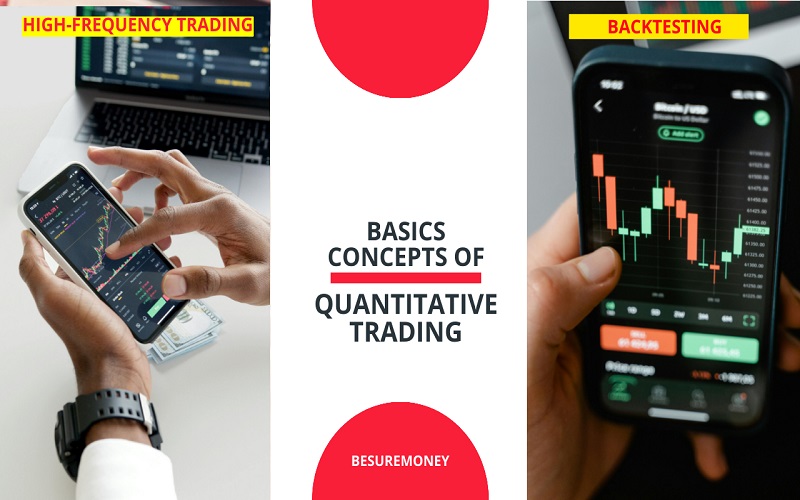
High-Frequency Trading:
It uses powerful computer programs. They transact many orders at very high speeds. Short periods don’t cause price changes in electronic financial markets.
Backtesting:
Backtesting is important in trading and investing. It evaluates the performance of a trading or funding method. It uses historical market data. It uses predetermined rules and limits to pass price data. This is to simulate how the strategy would have worked.
In quantitative trading, what types of data do traders use?
You can quantify quantitative data. You can calculate or measure it and assign a numerical value.
There are three types of quant data which have important information:
•Discrete
•Continuous
•Interval data
What are Quantitative Models?
Statistical models:
Statistical models are science approach that applies statistical analysis to data sets. A model shows a relationship between one or more random variables and others. Statistical models often represent the data generation process.
Machine learning model:
The machine learning model is a study in AI and computer science to recognize your patterns or data. Machine learning is a study of algorithms that help to train to find connections and patterns in data.
How does quantitative trading work?
It works by using data-driven to predict the probability of an outcome. In other forms of trading, it relies on accounting methods and systems to do this. Quant trading, when done well, can bring benefits. These include speed, accuracy, and the ability to analyze lots of data. Many hedge funds use quant trading strategies to make investment decisions. It is important to note that quant trade has its risks and challenges.
What are quantitative trading strategies?
Some strategies used to build mathematical models are:
Mean Reversion:
Mean reversion is a strategy based on math. It assumes prices will deviate from their “mean” in the short term and return in the long term. Markets are somewhat random. They cannot predict. So, we cannot emphasize this theory. Many historical examples involve forex, interest rates, and stocks.
Strategic development:
Strategic development requires you to consider both internal and external factors and to consider your business in that context, it is described as:
•Well-designed business strategies aimed at driving profitability.
•Develop a mitigation plan based on identified hazards, scenarios, and tradeoffs.
•Clear communication and implementation of policies through the use of defined action goals and success metrics.
•Understanding the values of strategies and their development can help you to grow your career as a manager.
Sentiment Analysis:
Sentiment Analysis is uncorrelated with market data, which at first may seem odd. However, in the age of the internet, many financial issues can affect the market. Sentiment analysis often uses natural language processing to analyze data. This can be in the form of social media, or research reports.
Challenges and risks of quantitative analysis:
Quantitative analysis is a cornerstone of decision-making across disciplines from economics to science. It uses mathematical and statistical techniques to analyze data and gain insights. Powerful quantitative research is not without its challenges. In this discussion, we will look at the challenges and risks of quantitative analysis.
Data quality and quantity:
One of the key challenges in this research is ensuring data quality and validity. The information provided can be incomplete, inaccurate, or biased leading to incorrect conclusions.
Also, getting enough relevant data is hard. This is especially true in niche or emerging areas with limited data.
Complexity and Interpretability:
While the analyses are comprehensive they are also complex. Defining complex models is hard. This makes it tough for stakeholders to understand and trust the results.
Balancing complexity and interpretability is a constant challenge in quantitative research. Simplifying models to make them clearer. This keeps them accurate and is easy to do.
Future Trends and Outlook:
Emerging Technologies:
Emerging technologies are constantly changing industries, societies, and economics, requiring intensive quantitative research to understand their impact, adoption rates, and likely future trajectories. Quantitative research in this area and the systematic collection and analysis of statistical data to gain insights into aspects of emerging technologies.
What are the advancements in technology for shaping futures in quantitative trading?
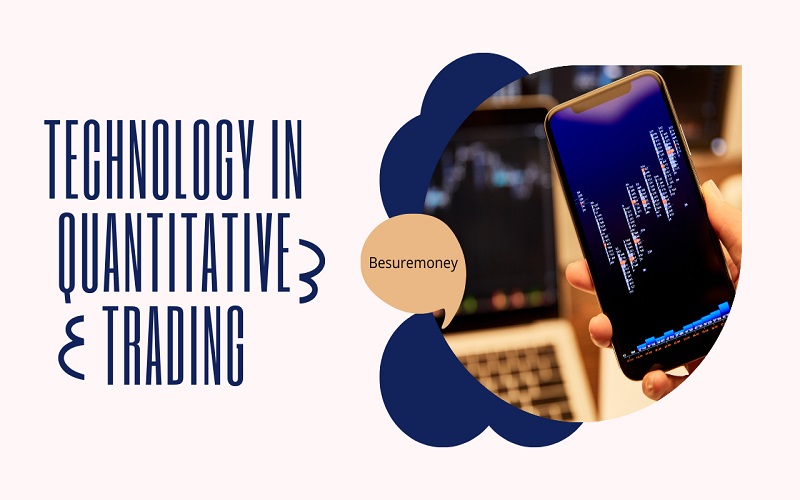
Trading was once dominated by human decisions and manual analysis. But, it has changed a lot with the arrival of technologies like AI, big data analytics, and blockchain. In this, we explore how this tech is reshaping big commerce.
AI in Quantitative Research:
AI, especially machine learning, has brought big changes to quantity trading. AI-powered trading systems can analyze lots of data. They can spot patterns and complexities that human traders can miss. These systems can adjust their strategies to trade well. They have learned to adapt to market changes.
Big Data Analytics:
The rise of big data has given quants a lot of hidden info. Traders can use advanced analytics to analyze huge data sets. These include market trends, social media sentiment, and macroeconomic indicators. This detailed view of markets helps traders make faster, data-driven decisions.
Summary:
Quantitative trading has increased the number of transactions in the financial markets. This is due to the strategic use of data and the potential benefits. It relies on statistical models. They analyze many types of data, like market trends and economic indicators. This model is the basis for quantitative trade strategies. These strategies range from statistical arbitrage to trend following. Yet, challenges such as algorithmic bias and market volatility remain. AI and big data are transforming manufacturing. They enable faster analytics and smarter choices. Despite the risks, this tech offers exciting possibilities. They are for the future of quantitative trading.
FAQs:
Q1. What mutual funds are managed entirely by quantitative trading algorithms?
Ans: This kind of algorithm governs all index funds. All passive funds are driven by observation and the number of observations plays an even greater role even with tight control. Then there is a difference between budgeting (allocation) and business process (execution).
Q2. Are quantitative trading firms embracing quantum computing?
Ans: Yes, some quantitative companies are exploring quantum computing to see how it can solve complex mathematical problems faster than traditional computers, but its widespread use is still in its infancy, as the technology is still developing and requires significant investment and expertise.
Q3. How important do you think backtesting is in a quant strategy?
Ans: Backtesting is important in quant strategy because it allows traders to use historical data to assess the feasibility and efficiency of their trading strategies and allows traders to identify strengths, weaknesses, and areas for improvement by how would method will work on performing in past market conditions. This helps them make more rational decisions and refine their strategies before deploying them to real-time offices.
Q4. How is quant trading used in crypto?
Ans: Quantitative trading in crypto makes use of mathematical models and algorithms to investigate marketplace information and execute the trades routinely. Traders use quantitative techniques to take benefit of patterns and developments inside the cryptocurrency marketplace, to make an income. These strategies vary from repetitive buying and selling to arbitrage. Given the volatility of the crypto market, buying and selling quantity can help traders navigate through rate fluctuations and exploit possibilities quickly and efficiently.